Automation in RCM: The Future of FQHC Billing
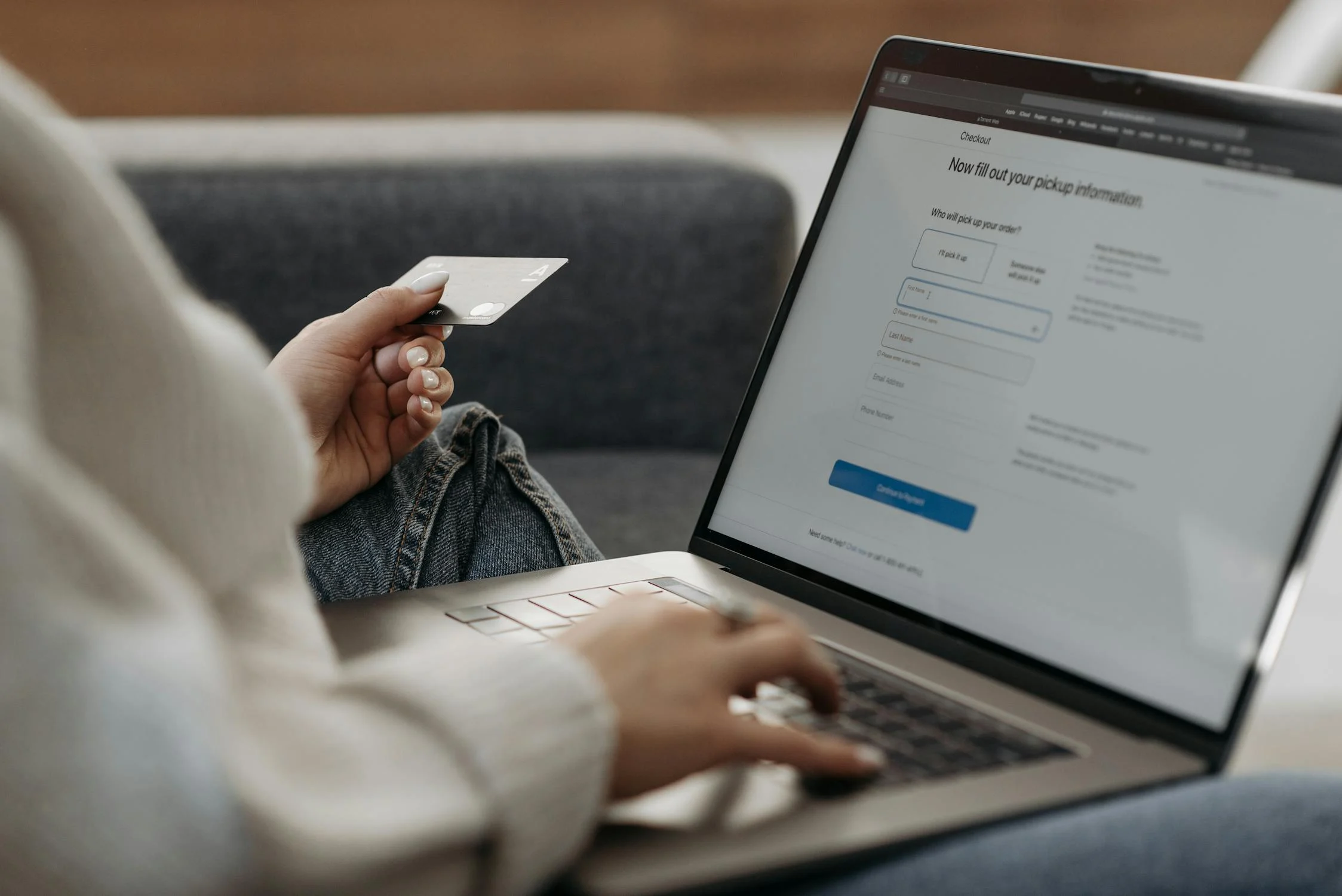
Introduction
Federally Qualified Health Centers (FQHCs) are vital to providing affordable healthcare to underserved communities, but their revenue cycle management (RCM) faces unique challenges. Manual billing processes, complex payer requirements, and limited resources often lead to claim denials, delayed reimbursements, and administrative burnout. These inefficiencies can jeopardize financial stability, with studies estimating that 15-25% of U.S. healthcare costs stem from administrative expenses, including billing. Automation, powered by artificial intelligence (AI), offers a transformative solution. By streamlining tasks like eligibility verification, claims processing, and denial management, AI-driven RCM reduces errors, accelerates payments, and frees staff to focus on patient care. For FQHCs, this means improved cash flow, compliance with regulations like the No Surprises Act, and enhanced patient satisfaction. Recent data shows automation can cut claim denials by up to 75% and reduce accounts receivable (A/R) days significantly. This article explores how AI automation is reshaping FQHC billing, highlighting key features and real-world examples to demonstrate its impact. The result? A sustainable financial future for FQHCs, ensuring they can continue their mission-driven work.
Section 1: AI-Powered Claims Processing
One of the most critical features of AI in RCM is its ability to optimize claims processing, a pain point for FQHCs where manual errors often lead to denials. AI tools use machine learning (ML) and natural language processing (NLP) to analyze claims before submission, flagging discrepancies such as incorrect coding or missing patient information. For instance, AI can cross-reference clinical documentation with Current Procedural Terminology (CPT) codes to ensure accuracy, reducing the likelihood of payer rejections. A 2023 Healthcare Financial Management Association (HFMA) survey found that 74% of healthcare organizations using AI for claims processing reported fewer denials, with some achieving a 40% reduction in rejection rates.
The process begins with automated claims scrubbing, where AI identifies errors in real-time, such as mismatched diagnoses or incomplete insurance details. Once corrected, claims are submitted electronically, cutting processing time from weeks to days. For FQHCs, which often juggle Medicaid, Medicare, and sliding-scale payments, this precision is invaluable. AI also adapts to payer-specific rules, ensuring compliance with diverse billing requirements. The benefits are clear: faster reimbursements, lower administrative costs, and reduced revenue leakage. By minimizing denials, FQHCs can stabilize cash flow, allocate resources to patient services, and avoid the costly rework associated with manual processes.
Beyond efficiency, AI-driven claims processing enhances transparency. Automated systems generate detailed reports on claim statuses, empowering FQHCs to track performance and identify bottlenecks. This data-driven approach not only improves financial outcomes but also builds trust with patients by ensuring accurate billing. As FQHCs face increasing patient volumes, AI’s scalability ensures they can handle growth without proportional increases in staffing costs, making it a cornerstone of modern RCM.
Section 2: Predictive Analytics for Denial Management
Another game-changing feature of AI in RCM is predictive analytics, which revolutionizes denial management—a persistent challenge for FQHCs. Denials, often caused by eligibility issues or coding errors, can delay payments by months, with the average cost to rework a denied claim estimated at $25-$50. AI-powered predictive analytics uses historical data to forecast which claims are at risk of denial, allowing FQHCs to address issues proactively. By analyzing patterns in payer behavior, patient demographics, and billing trends, AI identifies vulnerabilities before claims are submitted.
For example, predictive models can flag claims likely to be rejected due to lack of prior authorization or non-covered services, enabling staff to intervene early. A 2024 report by the American Hospital Association (AHA) highlighted that health systems using AI for denial management saw a 22% decrease in prior-authorization denials and an 18% reduction in denials for non-covered services. For FQHCs, where Medicaid denials are common due to complex eligibility rules, this capability is transformative. AI also prioritizes high-value claims for follow-up, optimizing staff time and improving collection rates.
The people aspect of predictive analytics is equally impactful. By automating routine denial analysis, AI frees RCM staff from repetitive tasks, reducing burnout—a critical issue given that 83% of healthcare leaders report labor shortages affecting RCM. Staff can focus on strategic tasks like patient engagement or negotiating payer contracts, enhancing job satisfaction and retention. The result is a leaner, more effective RCM team that drives financial stability. Predictive analytics also supports compliance by identifying trends that could trigger audits, ensuring FQHCs adhere to regulations like HIPAA and value-based care requirements.
Ultimately, predictive analytics delivers measurable benefits: shorter A/R cycles, higher first-pass claim rates, and increased revenue capture. For FQHCs, this translates to more predictable cash flow, enabling investments in community programs and infrastructure. By turning data into actionable insights, AI empowers FQHCs to navigate the complexities of billing with confidence.
Section 3: Real-World Examples
Real-world applications of AI in RCM illustrate its transformative potential for FQHCs. Consider Auburn Community Hospital, a 99-bed rural facility serving a diverse patient base similar to many FQHCs. Facing high denial rates and delayed billing, the hospital implemented AI-driven RCM tools, including robotic process automation (RPA) and NLP, nearly a decade ago. The results were striking: a 50% reduction in discharged-not-final-billed cases, a 40% increase in coder productivity, and a 4.6% rise in case mix index, reflecting more accurate coding. These improvements mirror the potential for FQHCs, where precise billing directly impacts funding for patient care.
Another example is Atlas Health, which partnered with an FQHC network to optimize financial assistance programs. Using AI, Atlas automated patient matching to eligible aid programs, analyzing demographics and medical needs in real-time. The system saved the FQHC 0.4-0.7% of net patient revenue annually, translating to thousands of dollars reinvested into services. This automation also improved patient satisfaction by simplifying billing, with fewer errors and clearer cost estimates—key to compliance with the No Surprises Act.
A third case comes from a California-based FQHC network that adopted AI for claims management. By deploying predictive analytics, the network reduced prior-authorization denials by 20% and accelerated payment cycles by 15 days on average. The AI system flagged claims at risk of rejection, allowing staff to correct issues before submission. This not only boosted revenue but also reduced administrative workload, enabling the FQHC to expand outreach programs without hiring additional staff.
These examples highlight tangible benefits: faster payments, lower costs, and enhanced patient experiences. Data from a 2024 McKinsey report reinforces this, estimating that AI and automation could save U.S. healthcare $200-$360 billion annually, with RCM as a key contributor. For FQHCs, these savings mean greater resilience in serving vulnerable populations, proving that AI is not just a luxury but a necessity for sustainable billing.
Conclusion
Automation in RCM, driven by AI, is redefining the future of FQHC billing. By leveraging features like AI-powered claims processing and predictive analytics, FQHCs can overcome longstanding challenges—denials, delays, and administrative overload. Real-world examples, from Auburn Community Hospital to Atlas Health, demonstrate that AI delivers measurable results: up to 75% fewer denials, 40% lower rejection rates, and millions in recovered revenue. These improvements translate to faster reimbursements, reduced costs, and more resources for patient care, ensuring FQHCs can fulfill their mission without financial strain. The data is clear—automation is not a trend but a critical tool for financial stability in a complex healthcare landscape. As FQHCs face growing patient demands and regulatory pressures, embracing AI-driven RCM is a strategic imperative. The path forward is simple: evaluate your RCM pain points, explore AI solutions tailored to FQHC needs, and take the first step toward a more efficient, patient-centered future.
Call to Action: Ready to transform your FQHC’s billing process? Assess your current RCM workflows and connect with AI-driven RCM providers to unlock efficiency and financial growth. Start today—your patients and bottom line deserve it.
References
- Healthcare Financial Management Association (HFMA), 2023 Survey
- American Hospital Association (AHA), 2024 Report
- McKinsey & Company, 2024 Healthcare Savings Estimate
- Thoughtful AI, 2025 RCM Automation Insights
No Spam —
Just Good Stuff.
Join our newsletter for actionable advice, insider knowledge, and strategies that drive real results.
No fluff, just value.
%20(1).png)