Alternative Payment Methodologies (APMs): Expanding Revenue Opportunities
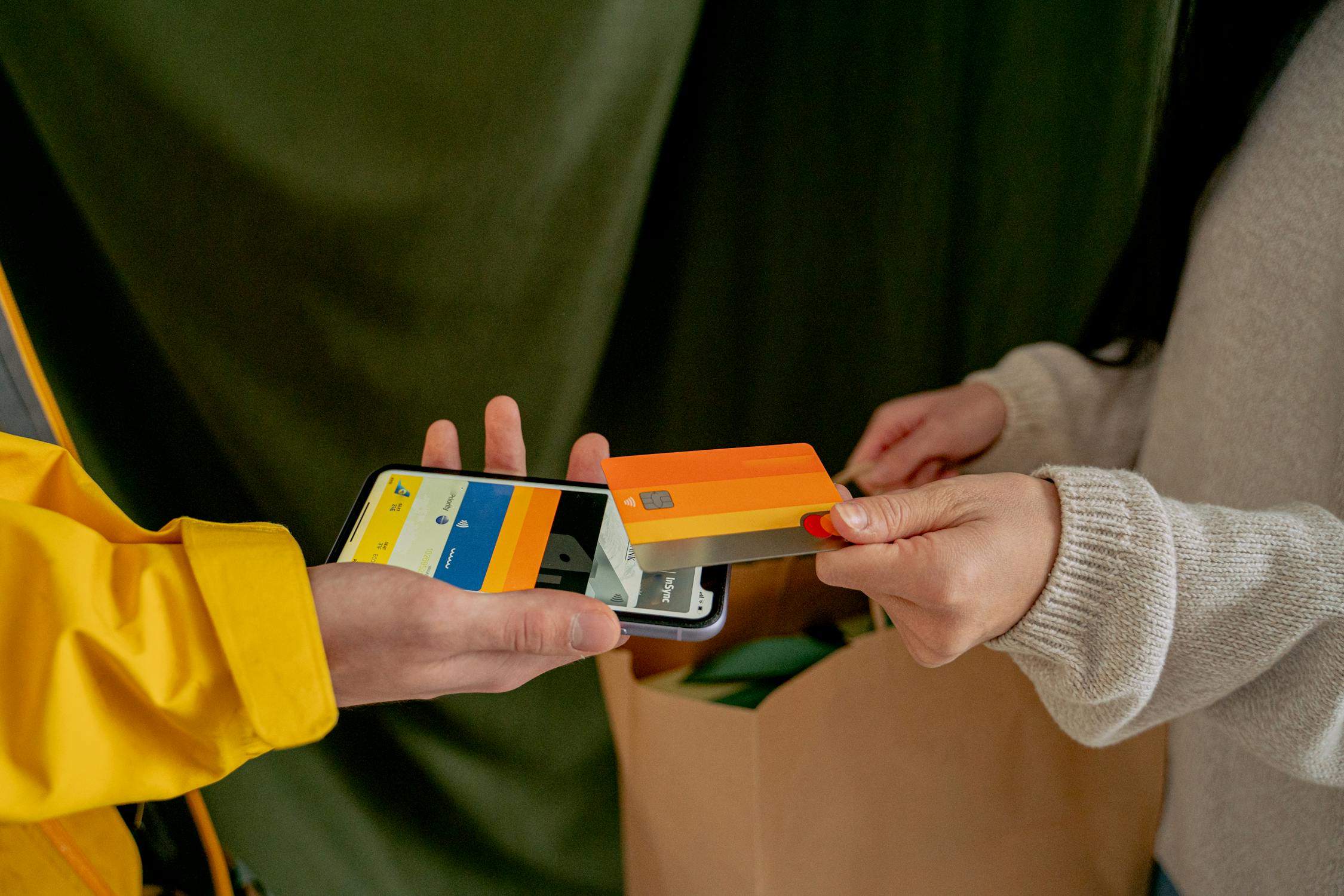
Introduction
Federally Qualified Health Centers (FQHCs) face financial pressures from traditional fee-for-service (FFS) models, which often fail to cover the full cost of care for underserved populations. In 2024, 65% of FQHCs reported revenue shortfalls due to reliance on FFS, limiting their ability to expand services (NACHC, 2024). Alternative Payment Methodologies (APMs), such as capitation, bundled payments, and value-based care, offer a solution by aligning incentives with quality and efficiency. Artificial intelligence (AI) enhances APM adoption by optimizing risk management and care coordination, boosting revenue by 10-20% and improving outcomes. Benefits include diversified income, reduced administrative burden, and stronger patient engagement. This article explores two AI-driven features—predictive risk stratification and automated APM analytics—supported by real-world examples. The result? FQHCs can tap new revenue streams, ensuring sustainability while advancing their mission of equitable care.
Section 1: Predictive Risk Stratification
A key feature for leveraging APMs is predictive risk stratification, a process that uses AI to identify high-risk patients and optimize care plans, aligning with value-based payment models. APMs reward FQHCs for preventing costly interventions, but identifying at-risk patients manually is labor-intensive and imprecise. AI analyzes electronic health record (EHR) data, social determinants of health (SDOH), and claims histories to stratify patients by risk level—low, medium, or high—for conditions like diabetes or heart disease.
For example, AI can flag patients with uncontrolled hypertension likely to require hospitalization, enabling early interventions like telehealth or community health worker support. A 2023 McKinsey study found that AI-driven risk stratification improved chronic disease management by 25% and reduced hospital admissions by 15% in value-based settings. For FQHCs, this aligns with capitation models, where fixed payments incentivize prevention. Predictive models also adjust for SDOH—like housing instability—ensuring equitable care, critical for Medicaid-heavy populations.
The people impact is significant. Clinicians, often overwhelmed (70% report burnout, AMA, 2024), receive prioritized patient lists, streamlining workflows. Care coordinators use AI insights to tailor outreach, boosting job satisfaction. A 2024 AHA study showed AI-supported teams increased patient engagement by 20%. Administrators benefit from data-driven grant applications, as funders prioritize outcome-focused FQHCs.
The result is clear: better outcomes, lower costs, and higher APM revenue. Risk stratification ensures FQHCs meet quality metrics—like 60% hypertension control—unlocking bonuses and shared savings, often millions annually, while enhancing community health.
Section 2: Automated APM Analytics
Another critical AI feature is automated APM analytics, a process that tracks performance and optimizes revenue under complex payment models. APMs require FQHCs to monitor diverse metrics—cost per patient, readmission rates, or preventive care uptake—across payers like Medicare and Medicaid. Manual tracking is error-prone, with 60% of FQHCs struggling to report APM data accurately (HFMA, 2024). AI automates this by integrating claims, EHR, and payer data into real-time dashboards, ensuring compliance with APM contracts.
For instance, AI can calculate savings in a bundled payment model for joint replacements, comparing costs to benchmarks instantly. A 2024 HIMSS study found that AI analytics improved APM performance tracking by 35% and boosted shared savings by 18%. For FQHCs, this means capturing revenue from value-based incentives, like $50-$100 per patient for meeting quality targets. Analytics also flag underperforming metrics—say, low cancer screening rates—prompting corrective action.
The people benefit is substantial. Administrators save hours on reporting, focusing on strategic growth; 68% of leaders using AI analytics report less stress (HFMA, 2024). Clinicians gain clarity on performance goals, fostering accountability. Billing staff align claims with APM requirements, reducing denials by 20%. This cohesion strengthens team morale, critical amid 78% of FQHCs facing staffing shortages (NACHC, 2024).
The outcome is transformative: maximized APM revenue, improved compliance, and scalable operations. Automated analytics empower FQHCs to thrive in capitation or shared savings models, diversifying income and funding expanded services like mental health or telehealth.
Section 3: Real-World Examples
Real-world cases illustrate AI’s role in expanding APM revenue. Unity Health Care, a Washington, D.C., FQHC serving 100,000 patients, adopted predictive risk stratification for a Medicaid capitation program. AI identified 5,000 high-risk patients with chronic conditions, triggering preventive care plans. Hospitalizations dropped by 20%, and Unity earned $2 million in shared savings in 2023. Patient satisfaction rose 15% due to proactive outreach, and staff reported a 25% workload reduction, showing how risk stratification boosts revenue and morale.
In Arizona, El Rio Health used automated APM analytics to excel in a Medicare value-based contract. AI tracked metrics like diabetes control (65% vs. 60% target) and cost efficiency, cutting reporting time by 40%. El Rio secured $1.5 million in bonuses, reinvesting in telehealth. Denials fell by 18% as analytics aligned claims with payer rules. Clinicians noted a 20% morale boost from clear performance goals, proving analytics’ dual financial and human impact.
A California FQHC consortium combined both AI features in a bundled payment model for maternity care. Predictive stratification reduced preterm births by 15% through targeted interventions, while analytics ensured cost savings met benchmarks. The consortium gained $2.2 million in revenue, with quality scores rising 22%. Staff saved 10 hours weekly, enabling community health expansion. These cases, backed by a 2024 NACHC report showing AI-driven APMs increased FQHC revenue by 10-20%, highlight clear benefits: millions in new funds, better care, and reduced burnout.
Conclusion
APMs offer FQHCs a path to financial resilience, and AI makes them accessible. Predictive risk stratification and automated APM analytics drive 10-20% revenue gains, cut hospitalizations by 15%, and save staff hours. Real-world successes—Unity’s $2 million savings, El Rio’s $1.5 million bonuses, and a California consortium’s $2.2 million—prove AI’s power. These tools enhance outcomes, streamline operations, and boost morale, letting FQHCs focus on patients. As traditional funding wanes, APMs are no longer optional. FQHCs must leverage AI to unlock their potential and secure a sustainable future.
Call to Action: Don’t miss out on APM revenue. Assess your FQHC’s payment model today and adopt AI-driven risk stratification and analytics to boost income and care quality. Start now to transform your financial outlook.
References
- National Association of Community Health Centers (NACHC), 2024 Report
- McKinsey & Company, 2023 Healthcare Outcomes Study
- American Hospital Association (AHA), 2024 Analytics Report
- Healthcare Financial Management Association (HFMA), 2024 Survey
- Healthcare Information and Management Systems Society (HIMSS), 2024 Study
- American Medical Association (AMA), 2024 Burnout Report
No Spam —
Just Good Stuff.
Join our newsletter for actionable advice, insider knowledge, and strategies that drive real results.
No fluff, just value.
%20(1).png)