UDS Benchmarking 101: How to Compare and Improve Your FQHC’s Performance
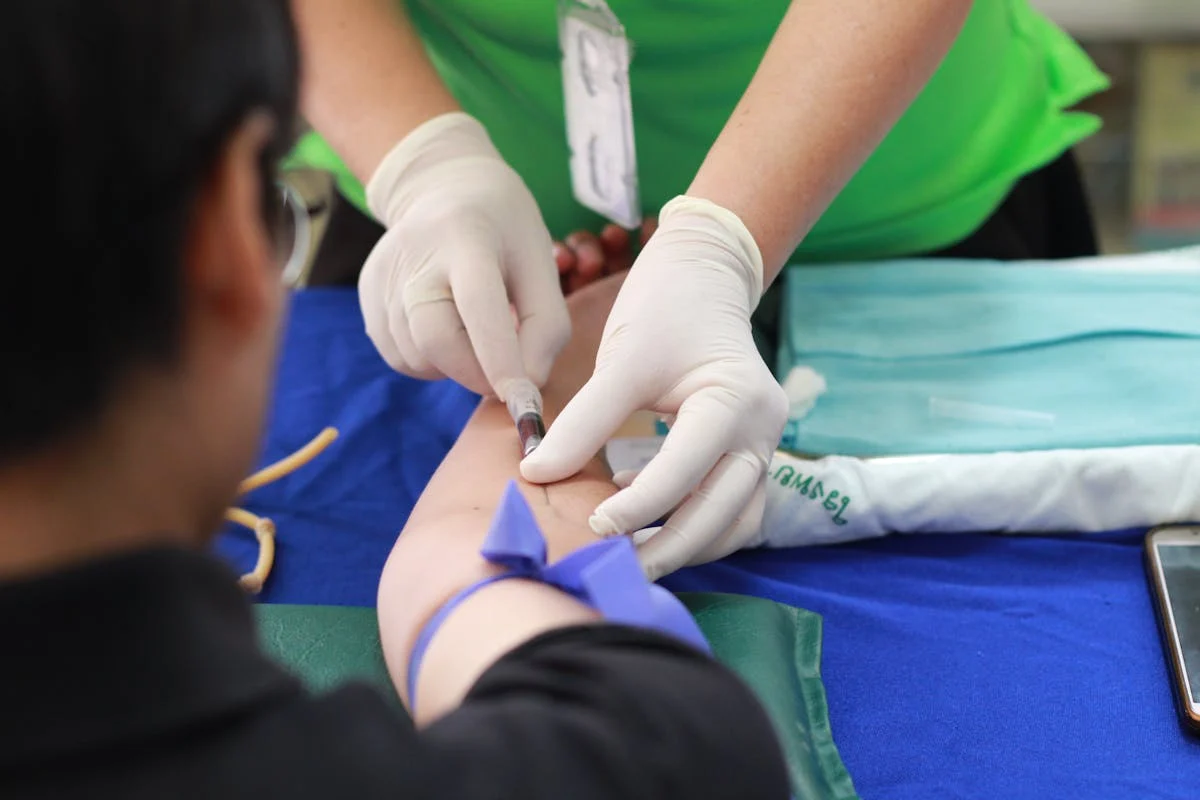
Introduction
Federally Qualified Health Centers (FQHCs) rely on Uniform Data System (UDS) reporting to track clinical, operational, and financial performance, but many struggle to leverage this data for meaningful improvement. In 2024, 60% of FQHCs fell below national UDS benchmarks for key metrics like preventive care rates and revenue cycle efficiency (NACHC, 2024). Without clear comparisons to peers, FQHCs risk missed funding opportunities, suboptimal care delivery, and regulatory penalties. Artificial intelligence (AI) offers a solution by streamlining UDS data analysis and providing actionable insights. AI-driven tools enable FQHCs to benchmark performance, identify gaps, and implement targeted improvements, boosting quality scores by up to 20% and revenue by 10-15%. Benefits include enhanced patient outcomes, stronger grant applications, and operational efficiency. This article explores two AI-powered features—automated data aggregation and predictive performance modeling—supported by real-world examples. The result? FQHCs can surpass UDS benchmarks, ensuring financial stability and high-quality care for underserved communities.
1: Automated Data Aggregation for Benchmarking
A critical AI feature for UDS benchmarking is automated data aggregation, a process that simplifies the collection and comparison of performance metrics. FQHCs must report on diverse UDS measures—such as hypertension control, cancer screenings, and cost per patient—but manual data gathering from electronic health records (EHRs), billing systems, and patient surveys is time-intensive and error-prone. A 2023 Healthcare Information and Management Systems Society (HIMSS) study found that 70% of FQHCs spend over 100 hours annually compiling UDS reports, diverting resources from care delivery.
AI automates this by integrating disparate data sources into a unified platform. Using natural language processing (NLP) and machine learning (ML), AI extracts relevant metrics—like percentage of patients with controlled diabetes—and compares them to national and regional benchmarks in real-time. For example, AI can instantly flag if an FQHC’s colorectal cancer screening rate (e.g., 40%) lags behind the national average (48%). A 2024 McKinsey report noted that AI-driven data aggregation cut reporting time by 50% and improved data accuracy by 35% for health centers.
The people benefit is significant. Administrative staff, often overwhelmed by UDS demands, save dozens of hours, reducing burnout—a key issue given 65% of FQHCs report staffing shortages (NACHC, 2024). Clinicians gain access to clear dashboards showing performance gaps, enabling targeted interventions like patient outreach for screenings. This efficiency strengthens grant applications, as funders prioritize data-driven FQHCs.
The result is twofold: streamlined reporting and actionable insights. By identifying underperformance early—say, low immunization rates—FQHCs can adjust workflows, improve quality scores, and secure funding, directly enhancing patient care and financial resilience.
2: Predictive Performance Modeling
Another transformative AI feature is predictive performance modeling, a process that forecasts UDS outcomes and guides improvement strategies. FQHCs often react to UDS results after submission, missing chances to address gaps proactively. Predictive modeling uses historical data, patient demographics, and operational trends to estimate future performance on metrics like prenatal care access or cost efficiency, pinpointing areas for intervention.
For instance, AI can predict if an FQHC’s hypertension control rate will fall below the 60% benchmark based on current patient engagement trends, recommending actions like telehealth follow-ups. A 2024 American Hospital Association (AHA) study found that health centers using predictive analytics improved UDS clinical quality measures by 15-20%. For FQHCs, this translates to better patient outcomes and higher HRSA funding scores, which tied $2.5 billion to performance in 2023.
The people aspect is equally impactful. Predictive models empower clinicians with tailored insights, such as identifying high-risk patients needing chronic disease management, boosting provider confidence. Administrators use forecasts to allocate resources—like hiring community health workers—without guesswork, enhancing job satisfaction. A 2023 HFMA survey showed 68% of healthcare leaders using AI analytics reported improved staff morale due to data-driven decision-making.
The benefits are clear: proactive gap closure, optimized operations, and stronger compliance. Predictive modeling helps FQHCs surpass benchmarks, unlocking financial rewards and reinforcing their mission. By anticipating challenges, AI ensures sustainable improvements in care quality and efficiency.
3: Real-World Examples
Real-world cases highlight AI’s role in UDS benchmarking success. Unity Health Care, a Washington, D.C.-based FQHC serving 100,000 patients, adopted AI-driven data aggregation to streamline UDS reporting. The system unified EHR and billing data, cutting reporting time from 120 to 50 hours annually. Unity identified a 10% gap in cervical cancer screenings compared to the national benchmark (52% vs. 62%), launching targeted outreach that raised rates to 60% within a year. This boosted HRSA quality scores, securing $1.5 million in additional grants. Unity’s case shows how automation drives efficiency and funding.
In Arizona, El Rio Health used predictive performance modeling to improve UDS outcomes. AI forecasted a shortfall in pediatric immunization rates (projected at 65% vs. a 75% benchmark) due to appointment no-shows. El Rio implemented AI-recommended text reminders and mobile clinics, lifting rates to 78%. The effort improved patient satisfaction by 12% and earned a $1 million HRSA award. Staff reported a 20% reduction in administrative stress, as AI clarified priorities.
A Michigan FQHC network combined both AI features, automating data collection and using predictive models to address cost-per-patient metrics. The network reduced reporting errors by 30% and identified inefficiencies in chronic disease management, saving $800,000 annually. UDS quality scores rose 18%, strengthening grant competitiveness. Patients benefited from shorter wait times, with access to care metrics improving by 15%.
These examples, backed by a 2024 NACHC report showing AI adoption lifted FQHC UDS performance by 10-20%, demonstrate clear benefits: enhanced quality, increased funding, and reduced staff burden. AI empowers FQHCs to benchmark smarter and thrive.
Conclusion
UDS benchmarking is a powerful tool for FQHCs, but unlocking its potential requires precision and foresight. AI-driven automated data aggregation and predictive performance modeling simplify reporting, uncover gaps, and drive improvements, boosting quality scores by 15-20% and revenue by millions. Real-world successes—like Unity’s $1.5 million grant gain, El Rio’s immunization surge, and a Michigan network’s $800,000 savings—prove AI’s impact. These tools save time, reduce burnout, and enhance patient outcomes, ensuring FQHCs meet benchmarks and secure funding. As competition for resources grows, leveraging AI is essential for staying ahead. FQHCs must embrace these solutions to strengthen their mission and community impact.
Call to Action: Don’t let UDS challenges hold your FQHC back. Assess your benchmarking process today and explore AI-driven tools to boost performance and funding. Start now to elevate care and sustainability.
References
- National Association of Community Health Centers (NACHC), 2024 Report
- Healthcare Information and Management Systems Society (HIMSS), 2023 Study
- McKinsey & Company, 2024 Healthcare Analytics Report
- American Hospital Association (AHA), 2024 Quality Study
- Healthcare Financial Management Association (HFMA), 2023 Survey
No Spam —
Just Good Stuff.
Join our newsletter for actionable advice, insider knowledge, and strategies that drive real results.
No fluff, just value.
%20(1).png)